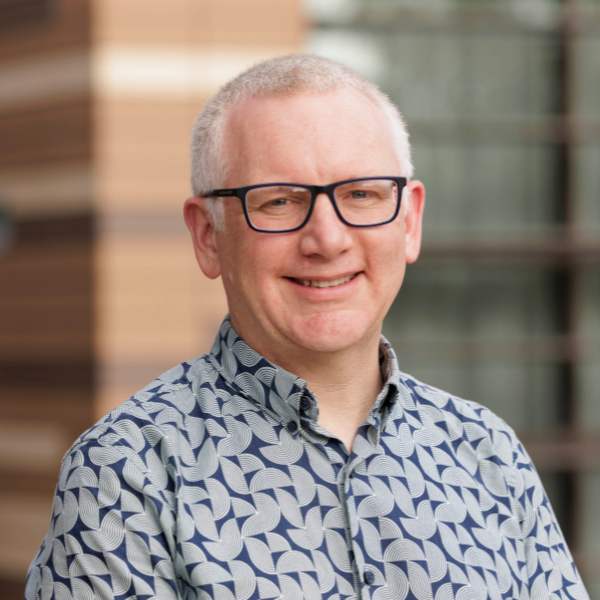
Establishing a causal relationship between an intervention and its effect on the population of interest requires two measures: an estimate of the outcomes for those who took part in the intervention; and an estimate of what would have happened in the counterfactual case – that is, the outcomes for those students if the intervention had not occurred.
Short of inventing a time machine and testing the same students with and without an intervention, researchers turn to methods that compare the outcomes between those who experience the intervention (the treatment group) and those who do not (the control group).
A reliable estimate of the intervention’s impact is critically dependent on how similar the individuals are in the treatment and control group. By similar, we mean that the members of each group are matched on observable variables (for example, age, sex and ethnicity) and unobservable variables (for example, motivation, wellbeing and interests).
Randomised controlled trials
The simplest way to ensure balance of observables and unobservables between a treatment group and control group is by random allocation from the population of interest prior to the intervention taking place. In large samples, these randomised controlled trials are usually the most robust way of estimating the causal impact of an intervention. Coupled with an implementation and process evaluation, this approach provides a clear picture of whether the intervention works, the context in which it works (or doesn’t) and for whom it works (or doesn’t).
Quasi-experimental methods
What to do if you can’t run a randomised controlled trial? You turn to quasi-experimental methods. These methods use statistical tricks to create a comparison group of individuals who did not take part in the intervention.
The most obvious starting place in constructing a comparison group is to select individuals from those who didn’t receive the intervention such that they match the intervention group on observable variables. Unsurprisingly, this process is called matching, and there are various methods to do this including propensity score matching, case control matching and exact matching.
Whichever matching method you use there is one major limitation. There is no way to ensure that your comparison group is matched to the intervention group on the unobservable variables. If the unobservable variables correlate positively or negatively with the outcome, they will confound any estimate of causal impact. For example, if the intervention is optional then students who do not take part may be less motivated than those who do and the comparator group will be mis-matched on the unobservable variables.
With this caveat in mind, you may be wondering if there are other quasi-experimental methods at your disposal which attempt to take into account the issue of unobservable variables. If you have sufficient historical outcome data prior to an intervention, you can use difference-in-differences or synthetic control methods. Alternatively, if a threshold is used to determine who receives the intervention (for example, in an income-contingent bursary) then a regression discontinuity design might be appropriate.
That is, if you can use these other quasi-experimental methods first then do so. But if they’re not feasible, matching may provide useful (albeit limited) evidence of an intervention’s efficacy.
A practical example: propensity score matching and case control matching
As part of our ‘Institutional data use’ project, Mike Kerrigan from Nottingham Trent University (NTU) provided a practical example of how to carry out propensity score matching and case control matching using the widely available statistical software, SPSS. The case study (PDF) shows how these methods can be used to estimate the impact of extra-curricular activities on academic performance at NTU.
In an appropriately caveated practical guide, Mike outlines the data prerequisites, explains how to perform matching using SPSS, and conducts an impact analysis. The impact of extra curricular activities on academic performance is found to be positively associated with academic performance. But, as Mike notes, this cannot be considered a causal evaluation since the matching of unobserved variables between the intervention and comparator groups is unknown.
Choosing an evaluation method
Our Data infrastructure guide includes a flowchart to help you choose the most appropriate evaluation method. Whatever method you choose, it is important to make the assumptions of the method clear. The degree to which you can meet those assumptions is an important part of contextualising the results.