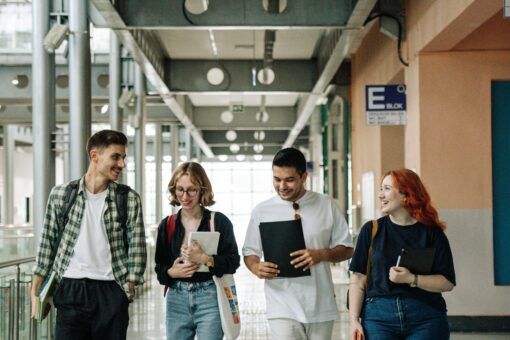
TASO was established with a clear mission: to bring robust evidence into higher education access and success. Central to this goal is generating new evidence on what works to achieve better outcomes for students from lower income backgrounds.
One approach to achieving this is through randomised controlled trials (RCTs), where some people are randomly assigned to receive a new intervention, while others are not. The random assignment means the groups are likely to be similar, and therefore any differences that emerge can be attributed to the intervention. This method is the easiest way to determine whether something works (or not), and is the foundation of evidence-based decision making across many fields, most prominently in medicine.
Challenges in higher education trials
In complex areas of policy such as higher education access and success, conducting a simple trial–where each person is randomised independently– is often not possible. Take, for example, a trial that we’ve been running to test the impact of informational booklets about bursaries on young people’s decisions about what university to attend.
The booklets are sent to schools and even if we addressed them to specific students l, there’s a decent chance that they’ll share the information with their friends, or that their teachers will make use of the resources we’ve provided. If the information isn’t shared, students who don’t receive booklets might interpret this as a signal – perhaps thinking they’re less capable than their peers or that universities aren’t so interested in them–depressing their likelihood of applying.
Here, we’ve got two potential sources of bias:contamination, where the control group starts behaving more like the treatment group, and reactance, where outcomes for the control group worsen because of their exclusion. . We can’t identify the magnitude of these biases, or even the direction of their cumulative effects. Therefore, a trial that randomly assigns some students to receive the booklets and others not to will give us a largely useless estimate of the effect of the intervention.
Randomisation at the cluster level
In this context, the solution is clear: randomise at the level of the school, so that all students in treatment schools get the intervention, while nobody in the control schools does. This approach eliminates two potential sources of bias. However, because the observations aren’t independent within school, this method requires more participants overall than when individually randomising.
The extent to which this is the case depends on a measure called the intra-cluster-correlation (ICC). The ICC tells us how similar individuals within the same school are to each other in terms of the outcome being studied. Higher ICC values mean that individuals within a school are more alike, which reduces the amount of unique information we get from each additional person in that school. As a result, higher ICC values require larger sample sizes to ensure the study has enough statistical power to detect meaningful effects.
Efficient and ethical trial design depends on having a good sense of what the ICC is. If we underestimate the ICC (guess a number that is too small) our study will be underpowered by lack of participants, increasing the risk of false negatives. On the other hand, if we overestimate the ICC (guess a number that is too high), we run studies that are too large. This not only increases the cost of the trial and limits the number of studies we can afford to run, it is also unethical to subject too many people to experimentation.
A systematic approach to trial design
Working out the ICC is simple, but rarely done. Instead, researchers rely on experience and intuition to approximate the right number, introducing inefficiency and uncertainty to the way we do research. To address this, we’ve published a paper in the Journal of Widening Participation and Lifelong Learning that calculates ICC values for a variety of trial designs. This paper also provides additional parameters that are useful for trial design.
This work, funded by the Cabinet Office, is part of a series of outputs producing boring but important information about trial design. We hope we will start to see 2025 as the first year of a transition from research generation as a cottage industry towards a more systematic approach to manufacturing.
Read the report: Useful parameters for the statistical design of trials in widening participation